Input Frame
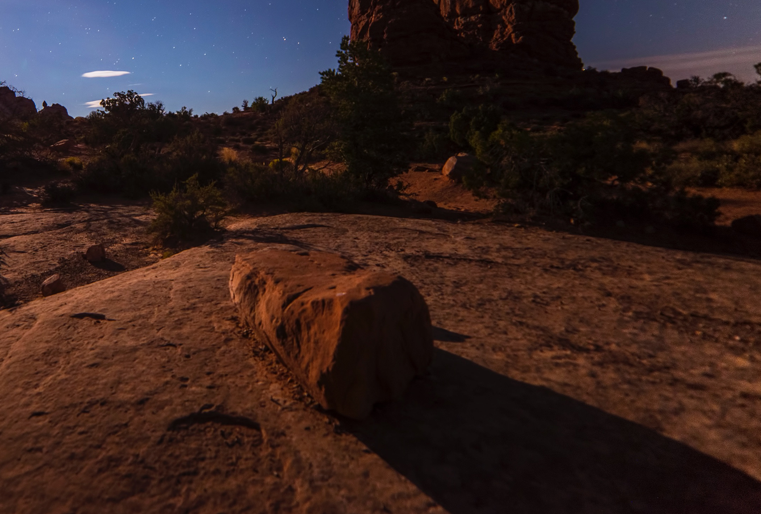
Real-world autonomous driving datasets comprise of images aggregated from different drives on the road. The ability to relight captured scenes to unseen lighting conditions, in a controllable manner, presents an opportunity to augment datasets with a richer variety of lighting con-ditions, similar to what would be encountered in the real-world.
This paper presents a novel image-based relighting pipeline, SIMBAR, that can work with a single image as input. To the best of our knowledge, there is no priorwork on scene relighting leveraging explicit geometric representations from a single image. We present qualitative comparisons with prior multi-view scene relighting base-lines.
To further validate and effectively quantify the benefit of leveraging SIMBAR for data augmentation for automated driving vision tasks, object detection and tracking experiments are conducted with a state-of-the-art method, a Multiple Object Tracking Accuracy (MOTA) of 93.3% is achieved with CenterTrack on SIMBAR-augmented KITTI - an impressive 9.0% relative improvement over the baseline MOTA of 85.6% with CenterTrack on original KITTI, both models trained from scratch and tested on Virtual KITTI.
(a) Geometry estimation: A single input image, I, is fed to monocular depth estimation networks (m). The predicted depth map, D, is used to form the vertex coordinates. The resulting set of vertices and faces forms 3D neural rendering mesh, M. (b) Image relighting: a set of input buffers, IB, are rendered with respect to the camera pose using M. The relighting networks refine the shadow maps to produce the relit output. Here, rsrc refines the source shadow map Ssrc and rtgt refines the target shadow map Ssrc. Finally, rout takes both as input, along with IB, to generate the final relit image.
To the best of our knowledge, there is no prior work on scene relighting leveraging explicit geometric representations from a single image. We compare our results with a prior multi-view scene relighting baseline – MVR.
We would like to highlight the qualitative results on the Virtual KITTI test set. The baseline CenterTrack model K has the greatest number of false positives and false negatives. Our model, trained with KITTI augmented with SIMBAR (K+S) has overall the best detection and tracking performance, and the least number of false positives and miss detections.
Quantitatively, we report that CenterTrack model trained on KITTI augmented with SIMBAR achieves the highest MOTA of 93.3% - a 9.0% relative improvement over the baseline MOTA of 85.6%. This model also achieves the MODA of 94.1% - again an impressive 8.9% relative improvement over the baseline MODA of 86.4%.